Text generators like ChatGPT have dominated my conversations with educators about AI in the past year. Despite their emergence a year before chatbots, text-to-image generators like DALL-E or Midjourney typically come up only in generic conversations about plagiarism or bias. This is understandable, both because writing has been central to so many disciplines and because text generators can produce impressive results quickly. Nevertheless, incorporating at least one exercise with AI image generators in a class can in a literal sense help students see the power and limitations of generative AI more clearly.
Pulling back the curtain on AI machinery
Open AI’s shrewd decision to frame ChatGPT as a chatbot with a single text field reinforces the impression that you are having a conversation with some kind of robot oracle. Triggering an image generator in a chatbot, like DALL-E inside ChatGPT, presents a similarly sanitized interface.
By contrast, the interface for a robust image engine like Leonardo.ai or DreamStudio.ai offers a panoply of options beyond simply what you type into the prompt box. Some are specific to image generation, like whether your image style should be 3d or anime or photorealistic, but others are parameters common to all deep learning networks that are hidden in most text-generating interfaces. (As of this writing, you and your students can burn through 150 credits daily before paying for image generation on Leonardo.ai, and there are similar free tiers for other generators based on the open-source Stable Diffusion engine.)
Showcasing output variability
Unlike most chatbot interfaces, almost every image generator cranks out a row of variations for a given prompt, essentially rolling the dice three or four times per request. Although they represent only a tiny fraction of the possible outputs for, say, a dog wearing a hat, seeing a corgi wearing a fedora emerge alongside a husky wearing a sombrero and probably some mongrels in between makes evident the probabilistic nature of AI content generation. It’s possible to produce multiple outputs by clicking the regenerate button in ChatGPT, but almost no one does, contributing to the misperception that there is only one correct answer for large language models to retrieve.
Demonstrating AI’s probabilistic character
An under-appreciated concept in generative AI is a model’s “temperature,” a measure of how far the output is allowed to stray from the most likely result. For a text generator, feeding “She stepped into my _______” into a transformer with a temperature of .3 might complete the sentence with “car” or “office”; increasing the temperature to .8 might produce less likely but also meaningful results like “dreams” or “life.”
Again, the temperature parameter is not typically adjustable for chatbots but can be set in apps like Leonardo.ai, so that “dog” might yield a labrador at low temperature but a coyote or fox at high temperatures.
Illustrating AI’s dependence on model
Under the hood, an app like GPT-4 consists of a number of models with different specialties that are invisible to the user. By contrast, a pull-down menu in Leonardo.ai lets users choose between models such as “PhotoReal,” “Pixel Art,” and “Pastel Anime Dream,” and the visual differences are striking.
Calling attention to AI fabrications
Both text transformers and image transformers churn out mistakes, but the latter are especially blatant. A History 101 student might be forgiven for confusing Richelieu with Robespierre, but the same student could easily blow the whistle on a picture of the Arc de Triomphe in Times Square or Napoleon with seven fingers on each hand.
Reminding students to prompt incrementally
For the reasons mentioned above, students are unlikely to be satisfied with an initial generation of AI-generated images. Users of image diffusion models quickly learn to play the field by repeatedly regenerating or refining their prompts. In a nod to their probabilistic nature, Midjourney calls this process “rerolling.”
The challenge of visualizing “averaged” language
Large language models represent words and word fragments as points in an abstract mathematical space. Unlike the three-dimensional space we inhabit, this space has billions of dimensions, so each point has billions of parameters rather than the usual x, y, and z. Each parameter for a given word can be thought of as the probability that another word will follow it in a sentence. Training an LLM on human-written sentences from the source data moves the points so that related words are clustered together.
When writing its responses, an LLM chooses the words that are most closely related to previous words from your prompt or its own replies. Depending on the temperature setting, these will be points near the middle of those word clusters. To find these mathematically, the LLM essentially averages the parameters of all the source words and builds a response from the words with the closest match to these parameters in the dataset.
Even though the sample datasets are huge—the Common Crawl has 3 billion web pages—there are plenty of unuttered but valid sentences that aren’t in the training data. As an analogy, the number 5 is the average of the numbers 2, 3, 7, and 8 even though it isn’t in the original set. Likewise, prompting an LLM with the right combination of familiar phrases can produce an “average” that is a completely new text. That’s why chatbots trained on comic books and Star Wars scripts can write a love story between Batman and Princess Leia that hasn’t existed previously.
Recognizing “averaged” imagery
Because images are more concrete than language, image generators make it much easier to see how generative AI fabricates averages from related points in its data set. Prompt for Queen Elizabeth at an Eminem concert or Johann Sebastian Bach winning a triathlon, and you’ll get a picture that pretty obviously has never existed before.
Despite their apparent originality, it’s not hard to point out how even exotic hybrids like these are mashups of stereotypes. Queens are likely to wear crowns and composers powdered wigs because that’s how we’re used to seeing them in popular depictions. And even for a hybrid, the “average” is skewed according to which constituent prevails in the training data; if the web contains more pictures of triathlons than composers, hybrids will look more like the former.
Asking for generic pictures of people is even more revealing. Early text-to-image models only returned white men when prompted to depict a professor or doctor. Generators like DALL-E have since improved the diversity of their outputs, but this isn’t because newer models have abandoned the stereotype generation at their core. Instead evidence has emerged of generators injecting hidden diversity terms to user prompts to improve the representation of women and people of color.
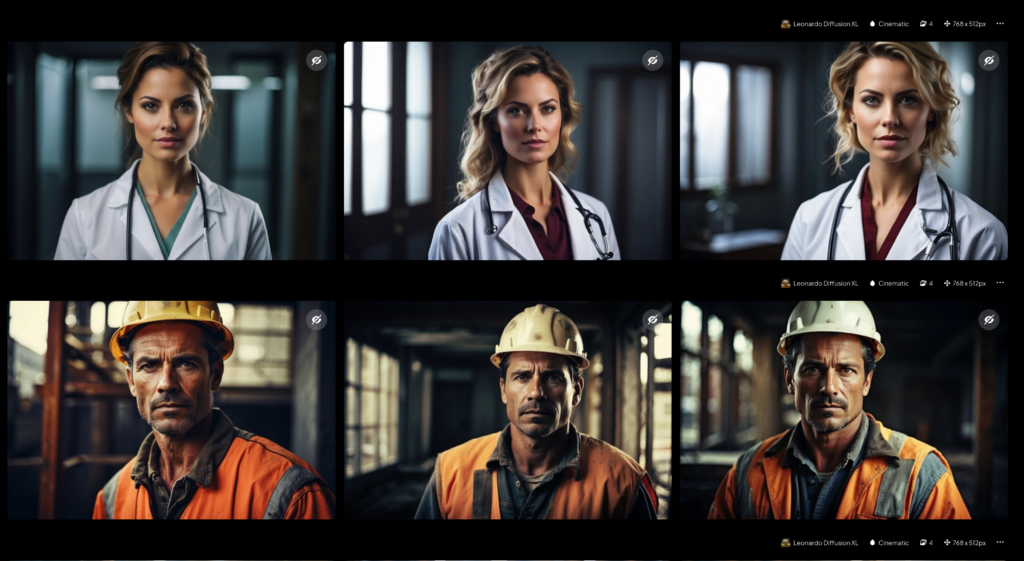
Identifying beauty stereotypes
Gender and ethnicity aside, it’s easy to point out to students how Midjourney and the like generate faces and figures that are stereotypically attractive, even without specific prompting. One cause is almost certainly a bias toward photogenic people in training data such as photos in Flickr and digital art in Deviant Art. Young people who’ve learned about the dangers of idealized body images in popular media may recognize its echo in AI images based on them.
A more insidious yet revealing cause has been dubbed the midpoint hottie problem. Studies suggest composite portraits–that is, averages of many individual faces–are more likely to be viewed as handsome or beautiful than one portrait chosen at random. One explanation is that composite photos “average out” abnormal proportions and eccentric features, resulting in a face that conforms to the uniformity and symmetry many associate with an attractive mate. Thus the prevalence of good looks in generative AI is another subtle but visible sign of an “averaging” dynamic that erases the margins, whether Black professors or people with crooked noses.
We might also redirect this realization back to text generators. ChatGPT and the like write remarkably grammatical and consistent prose, prompting some to wonder if LLMs understand syntax rather than just parroting it. But perhaps good grammar in this case is just “normal” in the mathematically sense of being average. Prose devoid of abnormality may sound desirable, of course, until we remember that writing without irregularities or idiosyncrasies is also writing without voice.
Understanding generative AI’s brand of creativity
Teachers with limited technical expertise or time to keep up with frenetic AI trends may feel intimidated by the prospect of tossing an image generation exercise into their syllabus. However, just giving students a chance to play with an image generator with minimal instruction is a quick way to deepen their grasp of how generative AI processes any task.
LLMs cannot be inspired to write a poem when they feel the sun on their face or to paint a storm cloud they saw in a dream. But they can leverage content in their dataset to create novel combinations if not novel content, which is why some of the more original outputs of text generators come from prompts like, “write the script for a romantic comedy that explains quantum mechanics.” Students might be more likely to try their hand at such hybrid genres if they see evidence that the average of two clichés isn’t necessarily a cliché. If you want them to grasp generative AI’s power along with its limitations, an image of the Pope wearing Balenciaga might be just do the trick.